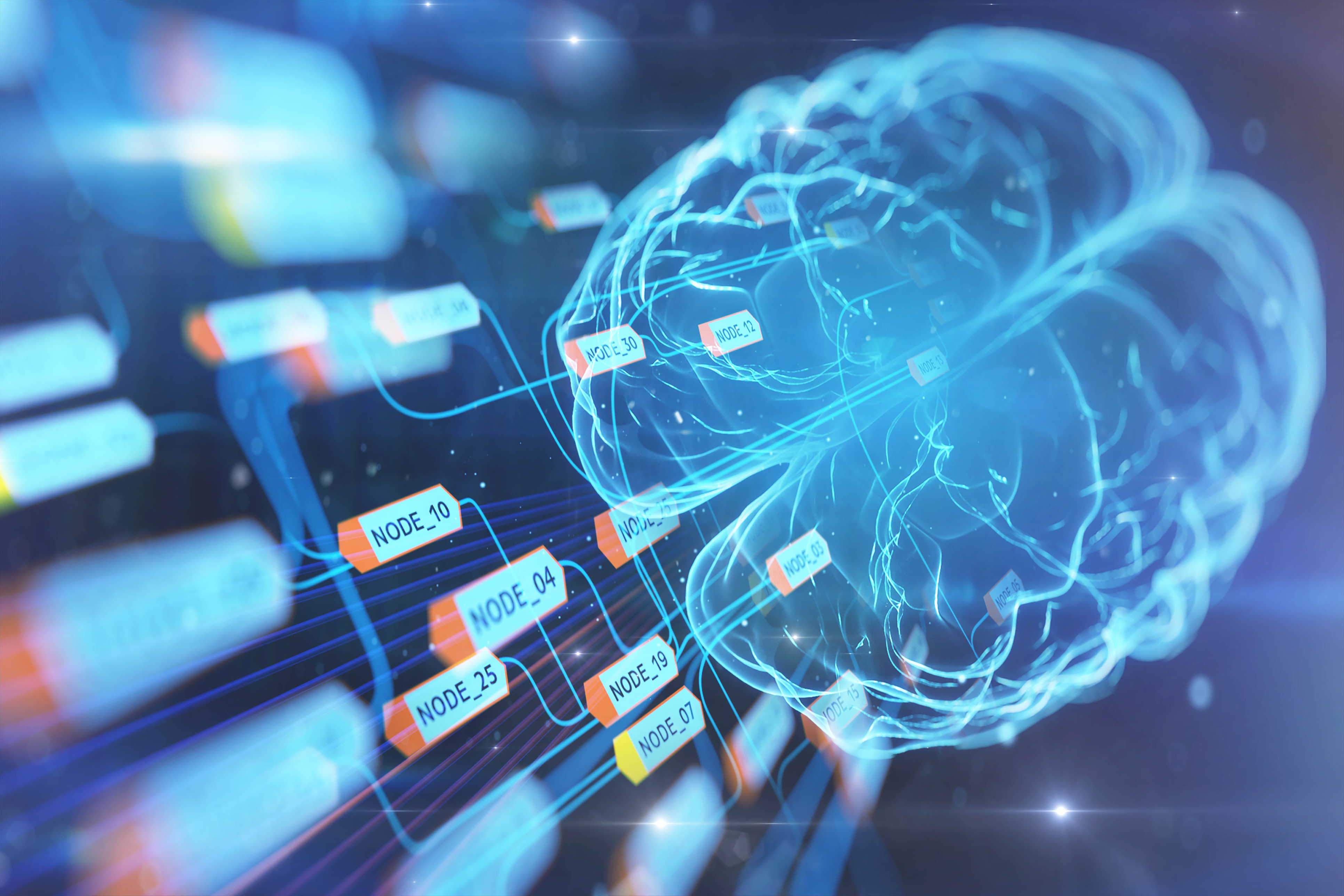
A day rarely ends without Artificial Intelligence (AI) and its vast benefit being discussed in some form or fashion. I am all for technological and mathematical advances; however, one needs to remember that we are early on this journey and we would be better served to avoid the “shiny object syndrome”, meaning chasing after an item merely because it catches our eye.
A recent study indicated that an AI algorithm contains bias. This finding is significant, not only because of the flaw it found, but also since it allows us to discuss both the positives of AI and the limitations. Statistical bias refers to when an algorithm produces a result that differs from the underlying estimate. This bias is pervasive in predictive analytics resulting from the small sample sizes, measurement error, and homogeneity/heterogeneity of the population that encompasses the baseline information. For example, as a hypertension specialist, I question the validity of using the Framingham calculator to predict the risk of a cardiovascular event in all populations. For instance, I stipulate that in the initial study, which is the basis for the predictive calculation that occurred in just one city, and most of the study participants were white males, an incredibly homogenous population. The predictive value is exceptionally high in a similar patient, but it under predicts risk by 20% for black individuals.
Social bias refers to inequity in care delivery that systematically leads to suboptimal care. Implicit and explicit biases complicate our present models of care and lead us to evaluate health equity issues. Once again, if this foundational problem exists, it leads us to have algorithms where there are flaws in the underlying data. Once again, the issue is not with the math; but with the underlying data that is used in the calculations. Possibly, machine learning may exacerbate this issue. For example; if a tool is using data from an electronic record, but the providers are delivering care in a biased way, the “learning” will continue to exaggerate the inequity, or effectively, the underlying implicit social bias. Missing data can also magnify these issues.
The root of these concerns is that the present quality of the data on which the machine learning trains is paramount for their success in truly advancing care. For us to move past this situation, we must address the data sources. First, are they representative of our populations? Secondly, do we need to review the possibilities for disparities before using the information? Finally, are there other data sources that allow the machine learning to correct for the first two? This latter point is critical for us to move forward. If we utilize multiple sources of information and ask the question, are biases evident in the data due to the reasons given? AI might even be able to help us identify such situations. This method would allow physicians to have tools that are both predictive in nature as well as identifying underlying care deliverer “human” conditions that might lead to implicit biases.
Unquestionably, AI is an enhancement that is here to stay; which means it is imperative that we create set standards for data usage and scientifically address these issues. The onus is on us as purchasers of this technology to ask these difficult questions of our vendors. They must answer these fundamental questions. Furthermore, I believe there is valid intent; however, we are extremely early in our understanding of AI. We must ensure we do not “fall in love with a shiny object’ without using our cognitive abilities to evaluate its underlying core information.