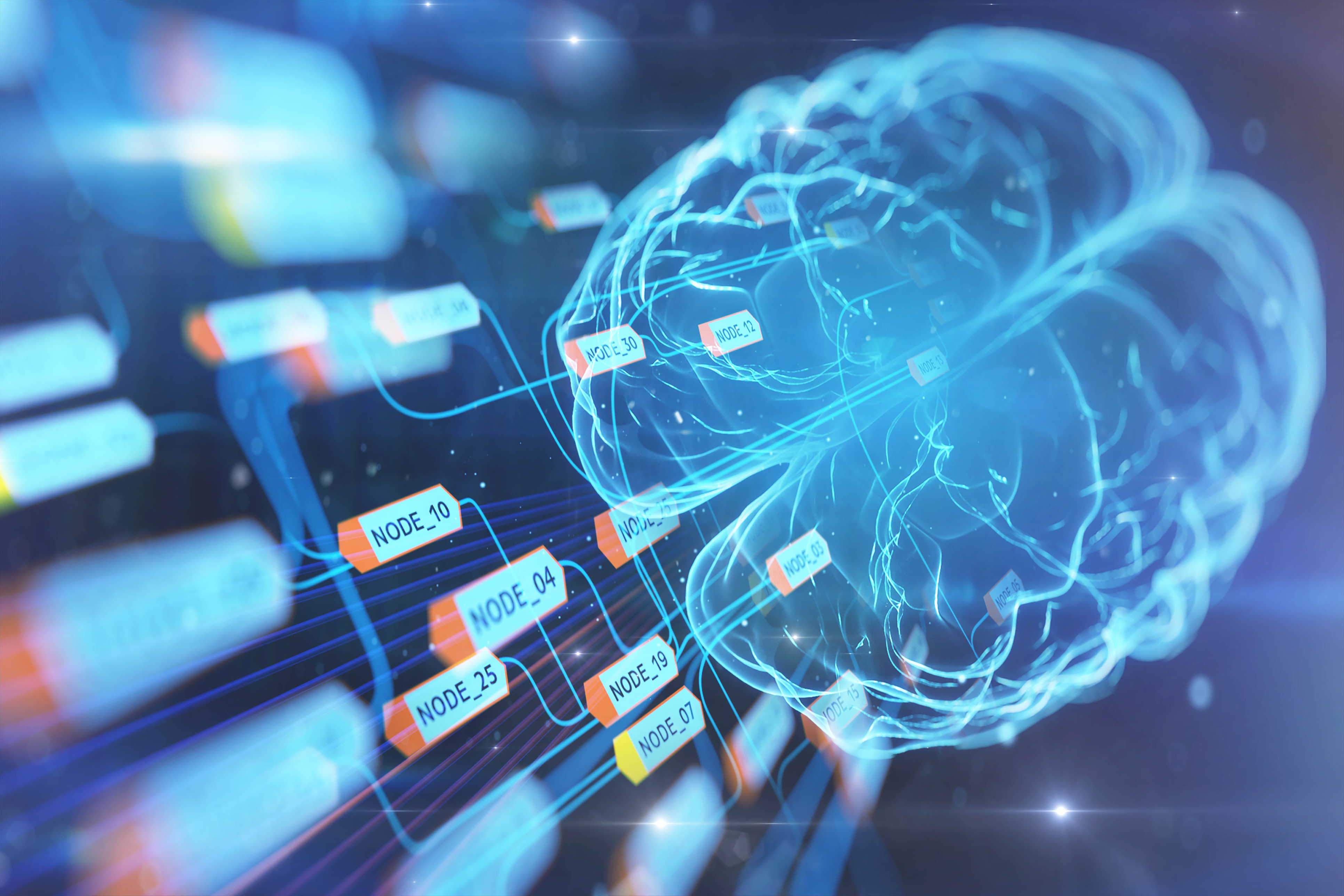
Unfortunately, to date, the utilization of artificial intelligence (AI) to improve and reduce inequities in access, quality, and appropriateness of high-quality diagnosis and care, has remained more of an aspiration than a reality. There are numerous reasons for this situation; the newness of AI, the low sensitivity and specificity of the algorithms, the lack of health equity data used in the algorithms, and the inability to implement results in current workflows to name a few.
As we progress on our journey to enhance our capabilities, especially within the AI space, we must consider an AI tool’s performance metrics. How do we measure success? Undoubtedly, how well the device works is critical, yet so is the clinician’s frequency of implementation, leading to a different diagnosis, treatment, or outcome. For example, the PhysioNet Challenge invites teams to develop algorithms. Their scoring includes high scores for the clinical utility of their predictions and low scores for misses and false alarms. This manner of assessment will improve the adoption of a tool because it focuses scoring on what matters to clinicians, thus allowing for better discernment of the value. Therefore, for this AI to be realistically adopted, we must measure it against clinically relevant metrics.
Additionally, as clinicians deliberate on whether to implement an AI tool or not, there are specific categories necessary to include in the decision-making. Evaluators should not assume that the historically utilized metrics fit the present situation. Naturally, AI is different; thus, thinking differently concerning the process is essential. Additionally, understanding the clinical involvement in the tool’s design is critical. Because of the nature of their jobs, data scientists have predominantly designed AI algorithms. However, they might miss the mark if they do not have the clinical context or the understanding of workflows.
Moreover, transparency of the method of “coding,” is imperative to both understanding the factors used, as well as being able to suggest adjustments. This need is vital to understanding if health equities may be addressed or not. These subgroup analyses will be crucial to improving our treatments based on more individual characteristics. Currently, we formulate our understanding based on studies done in limited populations to treat vastly different types of people.
To embrace AI, it must address both the needs of clinicians, and those they treat. Unequivocally, we must adjust our thinking to follow a different logic tree; however, we must base such thinking on enhanced capabilities. Otherwise, we risk implementing a tool that fails to improve outcomes. Unmistakably, AI is incredibly promising. Nonetheless, we must create the standards for its design and evaluation; otherwise, it will be thrown by the wayside prematurely.