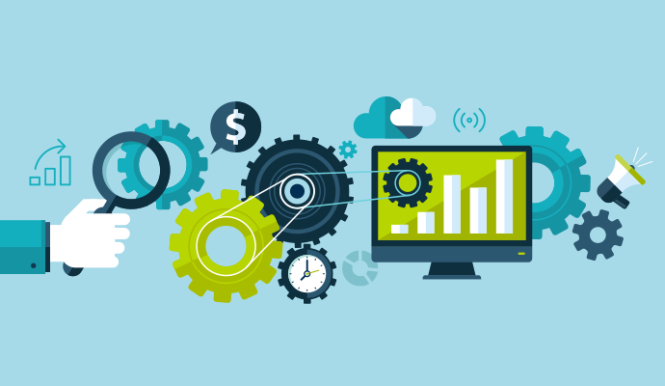
There has been considerable debate concerning the value of the Medicare Hospital Readmissions Reduction Program. The program was designed to deduct up to 3% of Medicare payments for hospitals with higher-than-expected thirty-day all-cause readmission rates, after index admissions, for six conditions and procedures.
One of the primary concerns regarding the program was the disproportionate impact on teaching and safety-net hospitals. The theory is that these hospitals are caring for a higher share of patients in poverty, patients with more severe social determinants of health, and those from racial or ethnic minority groups, would face more significant penalties.
As a result,, before attempting to solve the issue, they did not adjust the metrics for social risk during the calculations and these hospitals did have higher readmission penalties. The fear was that hospitals would begin to move away from caring for such patients because of the increased fines associated with such conditions. So, in 2019, the program changed to account for the association between social risk and higher admission rates.
A recent study in Health Affairs by Shashikumar et al. studied the impact of the 2019 changes on readmission rates. As hypothesized by CMS, they identified a reduction in penalties at those hospitals with the highest proportion of dual Medicare and Medicaid enrollees, rural hospitals, and those with a considerable share of Black and Hispanic or Latino patients.
These results demonstrate the need to stratify hospitals concerning both downside and upside value-based payment models. In doing so, providers are not penalized for serving those in segments of inequities; it also may act as a lever to decrease disparities.
Consequently, that change requires the metrics to account for such a situation’s impact change and allows adjustments to enhance the value of caring for such segments. This impact will create incentives to serve such populations and thus decrease the present disparities we encounter.
This programmatic change and resulting findings exemplify the importance of creating a learning environment and adjusting as programs mature. Instead of saying something is not working, the better question is, how do we improve on the present model? How do we reconfigure what we are presently doing to create our desired changes? Let us utilize these learnings and levers to ensure we are not disproportionally harming those we serve, and work to enhance the results we aim for those populations most impacted by the present inequities we have observed.