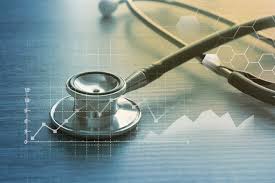
As generative Artificial Intelligence (AI) becomes increasingly integrated into healthcare, we must address its complexities and potential pitfalls. One of the most pressing concerns is the risk of bias, particularly when AI tools are used to support decision-making. While bias is not a new issue in medicine, it’s always been around. AI has the potential not only to exacerbate, but also significantly mitigate these biases, offering a hopeful and optimistic future for healthcare.
Medicine is built on a foundation of historical research and educational practices that are potentially fraught with bias. Additionally, implicit bias influences everyday clinical decisions, often without our conscious awareness. This bias may come from historical under representation in clinical studies, socioeconomic disparities, or even outdated training methods that fail to account for diverse patient populations. However, AI offers a unique opportunity to address bias much earlier in its use, unlike our traditional medical practice and learning models, where bias may be embedded.
Recently, The Department of Health and Human Services (HHS) Office (for Civil Rights issued a rule under Section 1557 of the Affordable Care Act that explicitly prohibits discriminatory outcomes from patient-decision tools, including AI. This ruling places a significant responsibility on healthcare providers to identify and actively mitigate bias when using AI-based systems, underscoring their crucial role in the ethical use of AI in healthcare.
This requirement presents both a challenge and an opportunity. On the one hand, there is a clear gap in how healthcare facilities can effectively implement and meet these new responsibilities. On the other hand, the heightened awareness of this responsibility can lead to closer scrutiny of the AI tools we use, ultimately benefiting the patients who rely on our care.
As purchasers and co-developers of AI technologies, healthcare providers are in a unique position to work hand-in-hand with AI developers in the creation of these algorithms. This collaboration is not just important; it’s essential. Moreover, we can, and should demand ongoing monitoring, greater transparency, and accountability from AI companies to ensure these tools meet the highest ethical standards. The era of proprietary algorithms cloaked in secrecy should come to an end.
Furthermore, it is crucial that other federal agencies align their oversight with the HHS’s recent ruling. Coordination among agencies like the Office of the National Coordinator for Health Information Technology (ONC), the US Food and Drug Agency (FDA), and HHS will be crucial. This alignment will provide clear and consistent guidance, reassuring healthcare providers and AI developers and instilling confidence in the regulatory process.
As with any new ruling, guidelines must be established for pertinent and necessary oversight. There is the opportunity to create a certification process for developers as well as a step-by-step understanding of how oversight and attestation are to occur. Other vendors are selling services to facilitate the monitoring of the requirements. However, this also underscores the core issue: healthcare organizations are being asked to fulfill regulatory mandates they may not fully understand, with the added burden of paying for third-party solutions that may or may not work. And every dollar spent on compliance is a dollar that could have been allocated to direct patient care.
To alleviate this burden, oversight agencies should develop and provide standardized testing tools to ensure consistent and straightforward implementation across the healthcare sector. Such tools would not only simplify compliance but also help ensure that healthcare dollars remain focused on patient care rather than administrative costs.
Ultimately, these new regulations are designed to protect the public. We have the opportunity to implement protections that not only enhance the desired outcome but also streamline the implementation of such tools in a manner that does not put additional strain on the healthcare ecosystem. This forward-looking approach can instill a sense of optimism about the future of AI in healthcare.