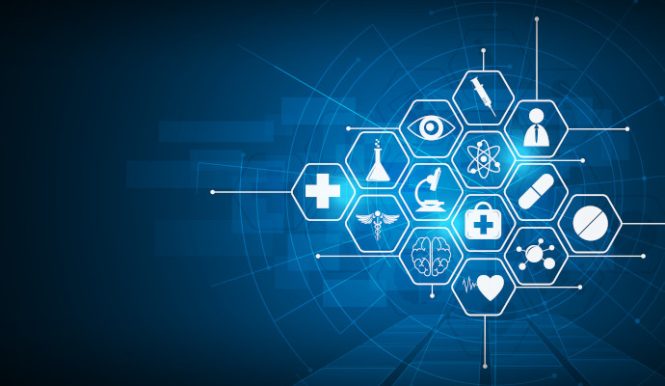
Currently, it seems Artificial Intelligence (AI) is at the forefront of everything, including influencing and transforming healthcare. At the same time, there continues to be a growing emphasis is on ensuring equitable access to healthcare services. Recognizing the potential biases within AI systems, the Department of Health and Human Services has taken a significant step by proposing rules to prevent discrimination in decision-making algorithms. This development signals a crucial moment to pause and work to eliminate historical biases ingrained within AI to harness its capabilities in addressing present health inequities.
First and foremost, to effectively address health disparities, it is imperative to understand the root causes. Historically, misconceptions about racial and ethnic differences have permeated healthcare, leading to flawed assumptions. These discrepancies range from overlooking physiologic variations to personal and implicit biases resulting in underdiagnosis and undertreatment. A proactive, race-aware approach to decision-making and support tools is essential in dismantling these ingrained disparities.
Solving these challenges requires a comprehensive strategy. Recognizing the problem and delving into its underlying causes is a crucial first step. Medical specialty societies can provide valuable guidance, and research funding sources can play a pivotal role by incorporating specific criteria that address trial design and participant inclusion. It is also critical to reshape algorithm development methods to prevent the exacerbation of health disparities and help enhance the ability to diagnose and treat equitably.
To find value in AI, collaboration with Public Health disciplines is paramount. AI companies can benefit from consultations with Public Health educators and researchers in university settings who understand the disparities and inequity we are trying to prevent. In fact, publicly available source codes can be integrated into algorithms, ensuring transparency and inclusivity. By leveraging relationships with Public Health experts, the AI community can enrich its understanding of diverse healthcare dynamics.
Mathematical approaches can also offer a systematic way to evaluate and adjust AI models. Assessing model performance across different population segments based on race, ethnicity, or social factors allows for identifying outcome discrepancies. The odds ratio can be balanced to correct algorithms, fostering more accurate and unbiased results.
Like the multifaceted strategies employed to improve health disparities in treatments and communications, this same approach is necessary for research and AI tools. Promoting education and awareness about these dynamics is crucial, as is addressing the root causes of biases. Establishing guidelines for research and AI development approaches will facilitate continuous evaluation, ensuring that the tools employed align with the goal of providing equitable solutions.
As AI revolutionizes healthcare, it is paramount to navigate its intersection with health equity consciously. By acknowledging historical biases, collaborating across disciplines, and employing diverse strategies, we can harness the potential of AI to help rectify existing health disparities and pave the way for a more equitable and inclusive future in healthcare. Before adopting new AI tools, it is essential to scrutinize vendors’ efforts in mitigating historical issues and aligning with the shared commitment to equitable solutions.